The Challenge of A/B Test Prioritization
Most experimentation teams generate far more test ideas than they could ever execute. This leads to a fundamental problem:
How do we objectively determine which tests to prioritize to maximize impact?
Over the years, various prioritization frameworks have been introduced to address this challenge. However, each has fallen short in critical ways, either relying too much on subjective human judgment or applying a rigid, one-size-fits-all approach that fails to adapt to different businesses, industries, and user behaviors.
But now, with the power of artificial intelligence, we believe we’ve found a near-optimal solution.
In this article, we’ll explore the limitations of traditional prioritization methods and introduce Confidence AI, our cutting-edge tool that overcomes these challenges to deliver smarter, data-driven test prioritization.
And for the skeptics out there, here’s a compelling reason to keep reading:
🔍 Confidence AI predicts winning A/B tests with 63% accuracy—several times more accurate than the average experimentation practitioner, based on standard industry win rates.*
*For details on how Confidence AI determines confidence scores, see the “What is Confidence AI and how does it work?” section.
Why Traditional Prioritization Frameworks Fall Short
Until now, leading test prioritization tools have consistently failed in two major ways:
1️⃣ Subjectivity – Experimentation is about making data-driven decisions, yet most frameworks rely on human intuition to estimate factors like “impact” and “confidence.” This injects bias and guesswork into a process meant to eliminate them.
2️⃣ One-Size-Fits-All Approach – Every website, page, and business operates in a unique context. Effective test prioritization requires a system that adapts to these differences, rather than applying the same rigid criteria across the board.
To illustrate these flaws, let’s look at two of the most commonly used prioritization frameworks: ICE and PXL.
The Limitations of ICE & PXL
ICE Framework (Impact, Confidence, Ease)
The ICE framework, popularized by GrowthHackers, scores test ideas based on:
- Impact – How big of an effect could this test have?
- Confidence – How sure are we that it will work?
- Ease – How simple is it to implement?
Each factor is rated from 1 to 10, and the average score determines prioritization.
🚨 The problem? These ratings are purely subjective—especially “impact” and “confidence,” which are impossible to accurately estimate before running a test. This makes ICE inherently unreliable.
PXL Framework (Weighted Scoring System)
The PXL framework, developed by CXL, aims to reduce subjectivity by introducing standardized scoring criteria believed to correlate with test impact. Each criterion is weighted, and tests are ranked based on their total score.
🚨 The problem? While it minimizes bias to some extent, PXL still lacks adaptability—it assumes the same factors predict impact across all industries, pages, and audiences, which is rarely the case.
Enter Confidence AI: A Smarter Way to Prioritize A/B Tests
Unlike traditional frameworks, Confidence AI removes subjectivity and adapts to your unique experimentation landscape. Instead of relying on human estimation, it leverages real-world data and machine learning to predict test outcomes with unprecedented accuracy.
In the next section, we’ll dive deeper into how Confidence AI works—and why it’s the future of A/B test prioritization.
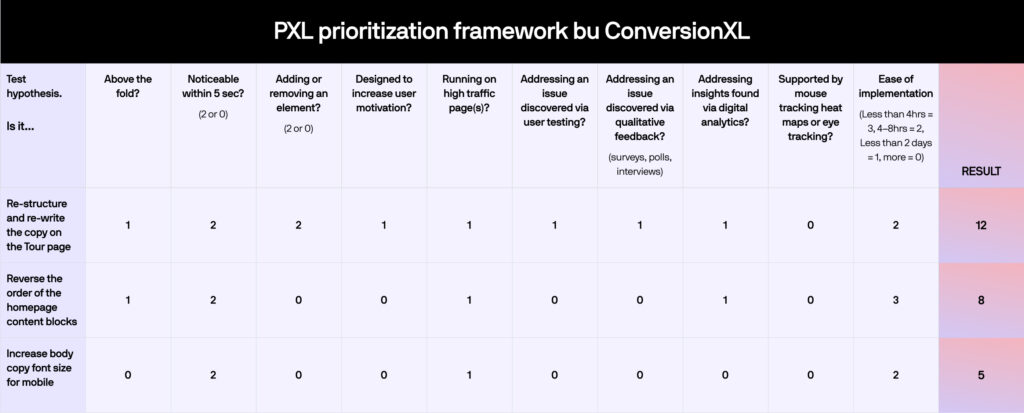
Why PXL Still Falls Short
While the PXL framework improves upon ICE by reducing subjectivity, it still suffers from the fundamental flaw of rigidity.
How PXL Reduces Subjectivity
PXL minimizes bias by incorporating more factual, yes/no criteria rather than subjective scoring. For example:
✅ Is the test above the fold?
✅ Does it involve adding or removing an element?
✅ Is the change noticeable within 5 seconds?
Because these are straightforward, objective questions, there’s less room for human interpretation, making the scoring process more reliable. Additionally, some of these questions can be answered using empirical methods—such as usability testing—rather than relying on gut instinct.
However, while this is a step in the right direction, PXL falls short in its assumption that the same criteria apply equally across all experiments.
The One-Size-Fits-All Problem
To see why this is an issue, let’s examine two key PXL criteria:
- “Above the fold?”
- “Noticeable within 5 seconds?”
For homepage experiments, these factors may strongly correlate with test impact. But for product pages, checkout flows, or deeper funnel pages, the same rules often don’t apply.
Users engaging with a product page are more willing to scroll, interact, and explore—meaning that the importance of “above the fold” placement varies drastically depending on context.
🚨 In fact, our own internal analysis shows that experiments below the fold often perform just as well—or even better—than those above it. (See graph below.)
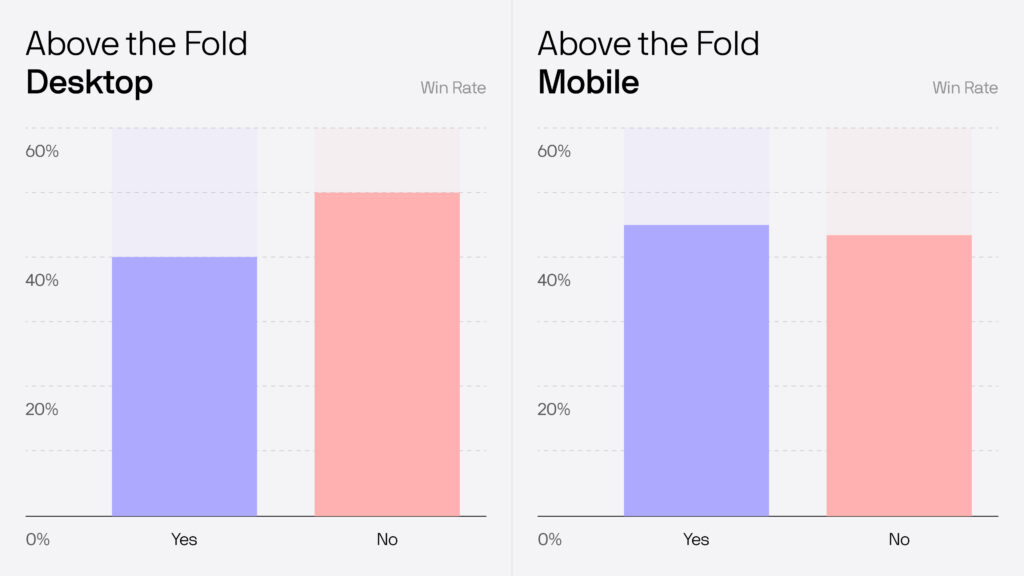
The assumption that “above the fold” tests are always more impactful simply doesn’t hold up. Our analysis of 505 A/B tests shows:
📱 Mobile:
- Above the fold win rate: 31%
- Below the fold win rate: 41%
💻 Desktop:
- Above the fold win rate: 37%
- Below the fold win rate: 36%
These findings highlight a critical flaw in traditional prioritization frameworks: they favor certain types of tests based on fixed criteria, rather than real-world data. This bias leads to suboptimal decision-making—some tests get prioritized simply because the framework is structured in their favor, not because they’re actually more likely to succeed.
So, while frameworks like ICE and PXL are useful, they fall far short of the ideal.
What Would an Ideal Prioritization System Look Like?
To truly optimize test prioritization, a system should:
✅ Be as objective as possible – Minimizing human bias in decision-making.
✅ Use flexible, dynamic criteria – Tailoring prioritization to the context of each test.
✅ Leverage past test results – Learning from historical data to improve future predictions.
✅ Require zero extra work – Integrating seamlessly into existing experimentation workflows.
✅ Continuously update priorities – Adjusting rankings dynamically based on new test results.
This last point is particularly important. Traditional prioritization methods typically freeze priorities at a single point in time—often at the beginning of a quarter or strategy cycle. This means the backlog quickly becomes outdated, failing to reflect the latest learnings from recent experiments.
Until now, building a system capable of meeting all these criteria was impossible.
But with Confidence AI, everything changes.
Introducing Confidence AI: Smarter Test Prioritization with Machine Learning
Confidence AI is a machine learning-powered prioritization system designed to predict the outcomes of A/B tests with unmatched accuracy. By embedding Confidence AI into the prioritization process, we eliminate subjectivity and rigid frameworks, replacing them with data-driven decision-making.
Here’s how it works:
🔹 Trained on 20,000+ experiments – Over the past 15 years, we’ve worked with 200+ clients across 30+ industries, collecting hundreds of thousands of data points on A/B test outcomes.
🔹 Context-aware predictions – Confidence AI analyzes test parameters—including industry, page type, change type, psychological principles, and more—to compute a confidence score predicting each test’s likelihood of success.
🔹 Seamless integration with Experiment OS – Within each client’s Experiment OS, Confidence AI automatically prioritizes experiments based on confidence scores. High-confidence tests move to the top, while low-confidence tests drop down the queue.
🔹 Dynamic, real-time updates – As new tests are run and fresh data is gathered, Confidence AI continuously refines its prioritization to reflect the latest learnings, ensuring your backlog is always up-to-date.
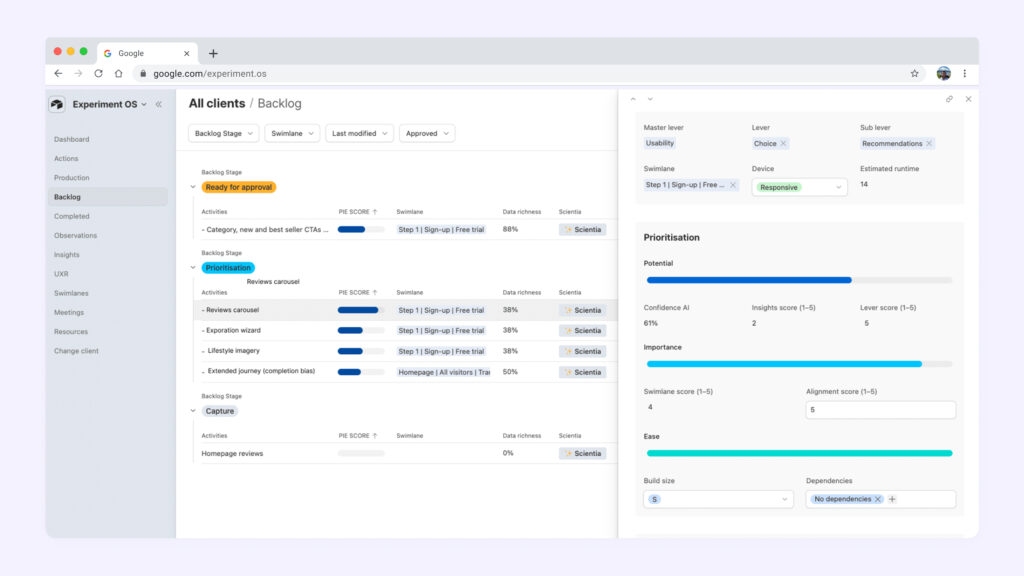
💡 The result? A smarter, more adaptive prioritization system that learns from past experiments and continuously optimizes for future success—without requiring any extra effort from your team.
With Confidence AI, prioritization is no longer a guessing game. It’s a data-driven strategy designed to maximize the impact of every test.
🚀 Ready to see it in action? Let’s dive deeper into how Confidence AI can transform your experimentation program.
How Accurate Is Confidence AI?
It’s one thing to theorize about a smarter way to prioritize A/B tests—but does Confidence AI actually work in practice?
To answer that, we put it to the test.
Putting Confidence AI to the Test
🔹 10 months ago, we rolled out Confidence AI across our entire consulting team.
🔹 Every time our consultants developed a new experiment concept, it was fed into Confidence AI to generate a confidence score (0-100) predicting its likelihood of success.
🔹 We then tracked the actual win rates of these experiments to see how well the model performed.
The Verdict? Confidence AI Works.
The data is clear: the higher the confidence score, the more likely a test is to win.
🔹 Experiments with high confidence scores (66-100) succeeded 63% of the time—more than double the industry average win rate.
🔹 Medium-confidence tests (33-66) still performed well, winning 43% of the time.
🔹 Low-confidence tests (0-33) won just 27% of the time, reinforcing the model’s ability to identify lower-probability experiments.
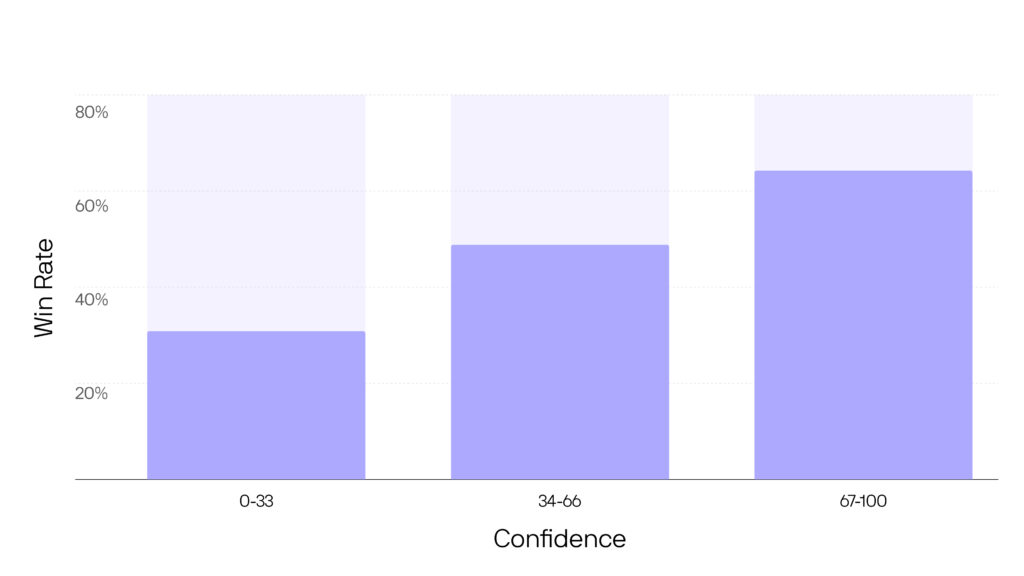
These results demonstrate that Confidence AI consistently predicts test success with remarkable accuracy, making it a game-changer for experiment prioritization.
🚀 The bottom line? Confidence AI takes the guesswork out of A/B testing—so you can focus on running experiments that actually move the needle.
Confidence AI vs. Industry Benchmarks: A Game-Changer in Experimentation
Elite experimentation teams at Microsoft, Airbnb, Google, and Booking.com report win rates between 8-30%—a far cry from the 63% accuracy Confidence AI achieves for high-confidence tests.
📌 If we assume that every experiment is launched with the expectation of winning, this means Confidence AI is dramatically outperforming the average practitioner in its ability to predict winners.
Why Are Industry Win Rates So Low?
It’s important to acknowledge that large-scale tech companies may have lower win rates for reasons unrelated to prediction accuracy, such as:
1️⃣ No-brainer changes (e.g., obvious UX fixes) are often implemented directly, skipping the A/B testing process.
2️⃣ Well-optimized websites mean incremental improvements are harder to achieve.
Despite these factors, we have never encountered an industry win rate that comes close to Confidence AI’s predictive accuracy, making it a truly valuable tool for prioritizing tests with the highest likelihood of success.
How We Actually Use Confidence AI (Beyond Win Rate)
Some of you may have noticed an interesting detail in our trial results:
🔹 49 high-confidence tests
🔹 120 medium-confidence tests
🔹 230 low-confidence tests
If Confidence AI is designed to prioritize high-confidence tests, why did we run so few of them?
This brings us to an important point: while win probability is crucial, it’s not the only factor in test selection.
The Balancing Act: Prioritizing for Speed, Resources, and Learning
When deciding which experiments to run, we also consider:
✅ Build complexity & resource constraints – A high-confidence test that requires a month of development and multiple approvals might be deprioritized in favor of a quicker, lower-confidence test.
✅ Velocity & iteration – Prioritizing tests that can be executed quickly allows us to gather data faster, feeding new learnings back into Confidence AI and improving future predictions.
✅ Exploring vs. Exploiting – While Confidence AI excels at identifying low-hanging fruit, experimentation is about more than just quick wins. It’s about exploring the full landscape of potential solutions to uncover global maxima—the most impactful optimizations over time.
The Bottom Line: Smarter, More Strategic Experimentation
With Confidence AI, we’re not just picking the highest-confidence tests—we’re building a holistic, strategic experimentation program that balances:
🔹 High win probability (maximizing ROI)
🔹 Execution speed (minimizing friction)
🔹 Iterative learning (continuously refining strategy)
🔹 Exploration (uncovering transformational insights)
This data-driven, adaptive approach is what makes Confidence AI more than just a prioritization tool—it’s a strategic experimentation engine. 🚀
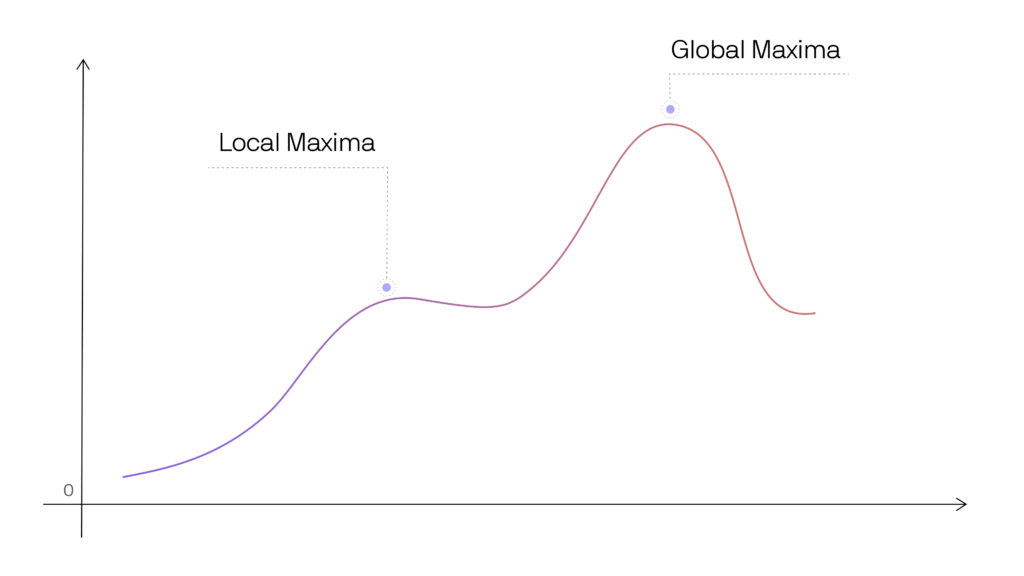
Confidence AI: A Powerful Tool, Not a Silver Bullet
Confidence AI is an incredibly powerful asset in our consultants’ toolkit. By providing a high-fidelity risk-reward assessment, it allows us to aggressively prioritize high-confidence tests that deliver strong short-term ROI for our clients.
But our mission as an agency extends beyond short-term wins—we focus on maximizing long-term ROI.
Beyond Incremental Gains: Experimentation as a Growth Engine
While safe, high-confidence tests are great for incremental improvements, the real breakthroughs come when experimentation is used as a strategic tool for bold innovation.
🚀 Confidence AI acts as a safety net, allowing clients to take calculated risks with greater confidence.
This approach is what enables us to help clients break through plateaus and push toward their true growth potential—beyond just small, safe optimizations.
That said, we recognize that each client has different priorities.
🔹 If a client wants to focus purely on short-term wins, we’ll leverage Confidence AI accordingly.
🔹 But most of our clients recognize the broader value of experimentation, so their programs emphasize exploration as much as exploitation—ensuring they don’t just optimize for the next quarter, but for long-term success.
Why We Don’t Just Prioritize High-Confidence Tests
Circling back to the question from earlier:
If Confidence AI is designed to prioritize high-confidence experiments, why don’t we always run more of them?
The answer is simple: the biggest gains often come from testing uncharted ideas.
While Confidence AI helps us identify and capitalize on clear opportunities, groundbreaking insights often emerge from experiments that challenge assumptions, explore new directions, and test bold hypotheses—areas where AI-driven predictions alone may not be enough.
Confidence AI: A Step Forward, Not a Magic Fix
There’s no question that Confidence AI has transformed how we prioritize tests, making our experimentation programs smarter and more efficient. But it’s not a silver bullet.
🔹 It’s only as good as the experiments we design – If the underlying research or execution is weak, Confidence AI’s predictive accuracy drops.
🔹 It prioritizes short-term impact, but long-term growth requires risk-taking – Confidence AI helps optimize, but it can’t replace visionary thinking.
🔹 The model is still evolving – This is just the first iteration, and as we gather more data, we expect its accuracy to continuously improve.
So, will there come a day when Confidence AI can predict every experiment outcome with unerring accuracy?
Maybe.
Until then, it remains a powerful tool—one that is only as effective as the experts using it. 🚀
Increase Revenue (CRO)
Want higher revenue from the traffic you’re already getting? Don’t pour more ad spend into a leaky funnel.
Get Started